Top Tips to Use Data Analytics in Customer Service
Article Summary:With the rapid advancement of technology, data analytics has emerged as a powerful tool for enhancing customer service effectiveness and efficiency. In this article, we will explore top tips for utilizing data analytics in customer service to optimize operations and elevate customer satisfaction.
Table of contents for this article
Effective customer service is essential for achieving success and cultivating loyal customers. With the rapid advancement of technology, data analytics has emerged as a powerful tool for enhancing customer service effectiveness and efficiency. Leveraging insights from data can provide valuable information about customer preferences, behavior patterns, and pain points, enabling businesses to tailor their services to meet evolving needs. In this article, we will explore top tips for utilizing data analytics in customer service to optimize operations and elevate customer satisfaction.
3 Steps to Implement Data Analytics
Understanding Your Current Landscape
Typically, for one business, assessing the current state of customer service within the business is crucial before delving into in-depth data analytics. You need to determine the importance of data analytics within your customer service team and clarify the objectives you aim to achieve through these insights.
This involves confirming key performance indicators (KPIs) such as response time, resolution rate, and customer satisfaction scores. Additionally, analyzing existing customer feedback and complaints to identify areas needing improvement is essential. By understanding the strengths and weaknesses of your customer service, you can establish a baseline to measure the impact of your data analysis initiatives.
Implementing an Insight System
To effectively harness the power of data analytics, it's crucial to have the right infrastructure in place. Implementing an Insight system, such as Udesk's Insight Analytics, can streamline data collection, analysis, and reporting processes.
These systems utilize advanced algorithms to aggregate data from various sources, including customer interactions, social media, and website traffic. By centralizing data within a unified platform, organizations can gain holistic insights into customer behavior and preferences.
Leveraging Insight Analytics
Once you have established an Insight system, it's time to leverage analytics tools to extract actionable insights.
If you want to monitor the performance of customer service team, you can create Custom Reports and Dynamic Dashboards, analyzing the workload and productivity of the team to ensure an equitable distribution of tasks.
If you want to anticipate customer needs and identify potential issues before they arise, analyzing historical data can help predict peak service demand periods, allowing you to allocate resources accordingly. Furthermore, sentiment analysis can provide valuable insights into customer emotions and perceptions, enabling personalized responses and proactive service delivery.
How Data Analytics can Improve Customer Service
Predictive Analytics for Proactive Support
Predictive analytics has revolutionized the way companies approach customer support, enabling them to anticipate and address issues before they arise. By harnessing the power of advanced algorithms and machine learning techniques, organizations can delve deep into their data to uncover hidden patterns and trends that signal potential challenges for customers. This proactive approach not only enhances the overall customer experience but also helps businesses mitigate risks and improve operational efficiency.
One of the key benefits of predictive analytics in proactive support is its ability to identify customers who are at risk of churning. By analyzing various data points such as purchase history, interaction frequency, and sentiment analysis from customer feedback, predictive models can accurately pinpoint individuals who exhibit behaviors indicative of potential churn. For example, a decline in engagement, coupled with negative sentiment expressed in customer interactions, may signal dissatisfaction and the likelihood of churn. Armed with this insight, companies can take preemptive action by reaching out to at-risk customers with targeted offers, personalized assistance, or proactive resolution of their issues, thereby increasing the likelihood of retention and loyalty.
In addition to identifying at-risk customers, predictive analytics can also optimize resource allocation and streamline support operations. By accurately forecasting demand based on historical data and anticipated trends, organizations can allocate resources more efficiently, ensuring that support teams are adequately staffed to handle incoming inquiries and requests. Furthermore, predictive models can help prioritize and triage support tickets based on their likelihood of escalation or impact on customer satisfaction. By directing resources to high-priority issues or customers with urgent needs, companies can improve response times, reduce resolution times, and enhance overall service quality.
Personalized Customer Experiences
Data analytics serves as the foundation for delivering these personalized experiences at scale. By leveraging a wealth of customer data, including demographics, browsing behavior, purchase history, and engagement patterns, companies can gain deep insights into individual preferences and interests. Armed with this information, businesses can create targeted marketing campaigns that resonate with each customer segment, delivering relevant content and offers that are tailored to their specific needs.
Moreover, data analytics enables companies to provide personalized product recommendations, guiding customers towards items that align with their preferences and past purchases. By analyzing historical data on product interactions and purchase patterns, organizations can use collaborative filtering algorithms and recommendation engines to suggest products that are likely to appeal to each individual customer. This personalized approach not only enhances the shopping experience but also increases the likelihood of conversion and repeat purchases, driving revenue and customer lifetime value.
Continuous Improvement Through Feedback Analysis
Feedback analysis is essential for driving continuous improvement in customer service and ensuring that businesses stay responsive to evolving customer needs and preferences. Data analytics plays a crucial role in this process, enabling companies to gather, analyze, and act upon feedback from various sources, including social media, surveys, and online reviews.
Natural language processing (NLP) and sentiment analysis techniques allow organizations to extract valuable insights from unstructured feedback data, such as customer comments and reviews. By analyzing the sentiment and themes expressed in this feedback, companies can identify common pain points, recurring issues, and areas for improvement. For example, sentiment analysis may reveal patterns of dissatisfaction related to product quality, shipping delays, or customer service responsiveness.
Insight System: The Best Tool of Data Analytics
Udesk Insight System is a comprehensive platform designed to facilitate the collection, analytics, and interpretation of data to derive actionable insights and make informed decisions. It integrates various technologies, methodologies, and tools to gather data from disparate sources, process it, and generate valuable insights that drive business strategy, innovation, and optimization.
Now it’s time to choose Udesk Insight System, it will bring you a surprised experience.
》》Click to start your free trial of Insight, and experience the advantages firsthand.
The article is original by Udesk, and when reprinted, the source must be indicated:https://www.udeskglobal.com/blog/top-tips-to-use-data-analytics-in-customer-service.html
InsightInsight AnalyticsInsight System
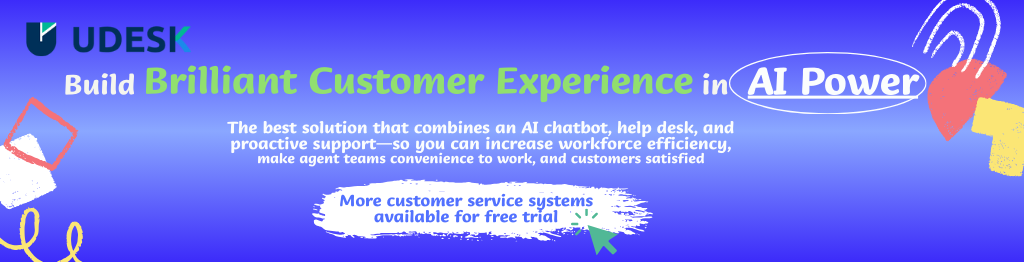